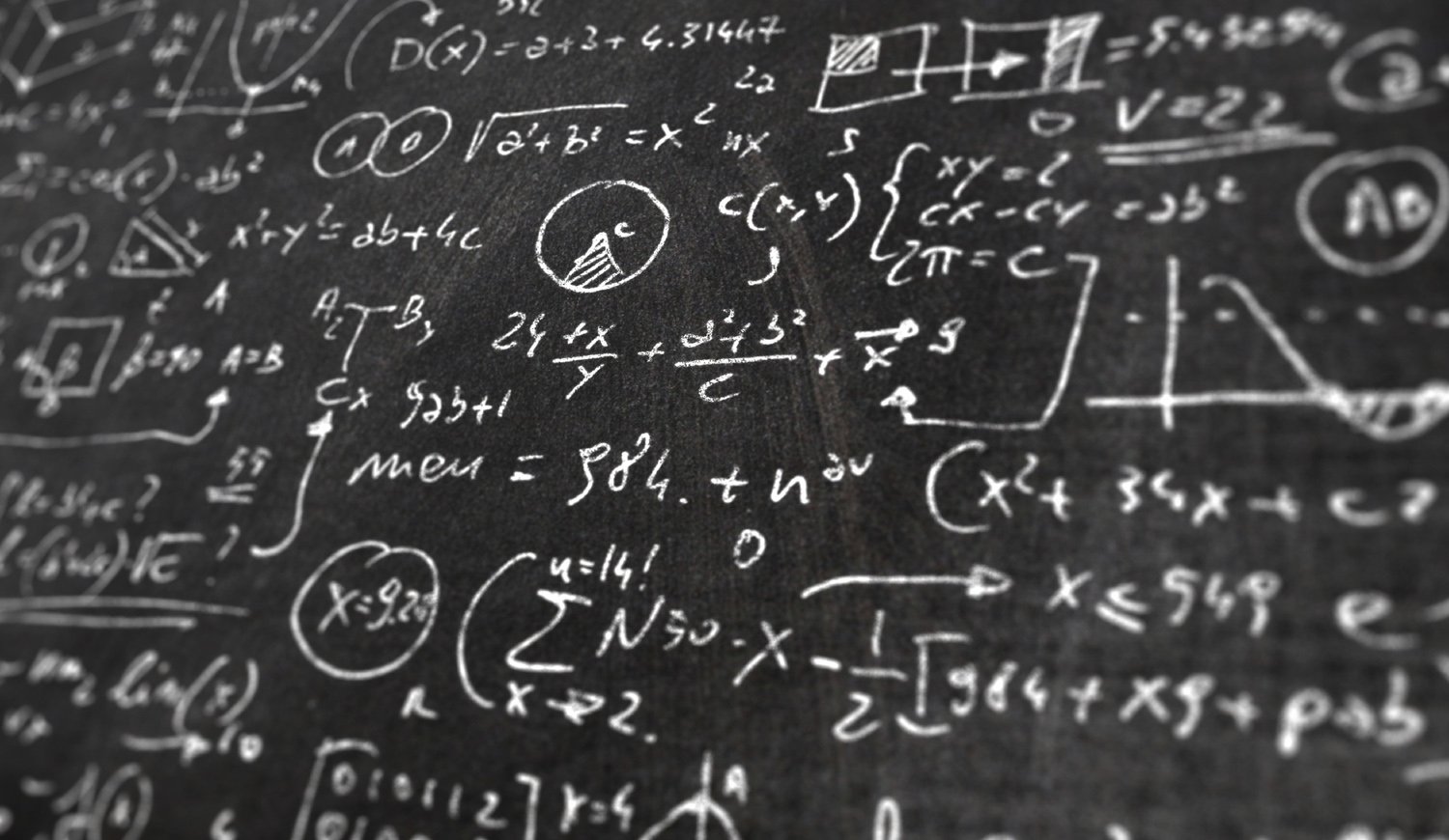
Summarising data
Researchers
Why is it important?
Summarising data is usually the fundamental basis to which the background data on a cohort is described. Often it is too cumbersome to describe all the features of each individual that is studied and becomes impossible to do so with large numbers.
How is it done?
In general data are summarised with two measures, a statement of “central tendency” followed by a statement of “variation”. In general data are divided into continuous (normal and non-normally distributed) or categorical (binary or multiple categories).
It is important to separate continuous data in normal and non-normally distributed because the summary measures used are different. When data is normally distributed (e.g. age, figure 1) we can use summary measures such as the mean and standard deviation. Because of the distribution of the data, we can use “shortcuts” to calculate the spread, for example we know that 95.45% of the observations will lie between 2 standard deviations of the mean. This is not the same for non-normally distributed data (e.g. length of follow up in years, figure 2), which is summarised as median and interquartile range.
Categorical data can be more straightforward. It can be a binary category (e.g. gender with only two outcomes, male or female) or multiple categories (e.g. colour). Categorical data is summarised as frequency and percentage e.g. 32 (34%).
What is the relevance?
If you apply the mean and standard deviation wrongly to describe the data that is not normally distributed, for example mean length of follow up of 4 years, standard deviation of 3 years, then the data is interpreted as 95.45% of the observations will lie between -2 years and 10 years. It is impossible to have a length of follow up of -2 years!
It is important to appreciate outcomes such as cancer stage (I-IV) should be treated as categorical and not continuous, because a cancer stage of III does not imply that the outcome is 3 fold worse than a cancer stage of I.
Normally distributed data
Non-normally distributed data
If you find this type of teaching useful and would like to learn more, I run an online statistics course for clinicians and researchers: